Copy Link
Add to Bookmark
Report
AIList Digest Volume 1 Issue 069
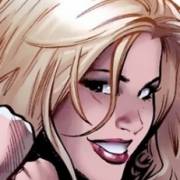
AIList Digest Monday, 3 Oct 1983 Volume 1 : Issue 69
Today's Topics:
Rational Psychology - Examples,
Organization - Reflexive Reasoning & Conciousness & Learning & Parallelism
----------------------------------------------------------------------
Date: Thu, 29 Sep 83 18:29:39 EDT
From: "John B. Black" <Black@YALE.ARPA>
Subject: "Rational Psychology"
Recently on this list, Pereira held up as a model for us all, Doyle's
"Rational Psychology" article in AI Magazine. Actually, I think what Pereira
is really requesting is a reduction of overblown claims and assertions with no
justification (e.g., "solutions" to the natural language problem). However,
since he raised the "rational psychology" issue I though I would comment on it.
I too read Doyle's article with interest (although it seemed essentially
the same as Don Norman's numerous calls for a theoretical psychology in the
early 1970s), but (like the editor of this list) I was wondering what the
referents were of the vague descriptions of "rational psychology." However,
Doyle does give some examples of what he means: mathematical logic and
decision theory, mathematical linguistics, and mathematical theories of
perception. Unfortunately, this list is rather disappointing because --
with the exception of the mathematical theories of perception -- they have
all proved to be misleading when actually applied to people's behavior.
Having a theoretical (or "rational" -- terrible name with all the wrong
connotations) psychology is certainly desirable, but it does have to make some
contact with the field it is a theory of. One of the problems here is that
the "calculus" of psychology has yet to be invented, so we don't have the tools
we need for the "Newtonian mechanics" of psychology. The latest mathematical
candidate was catastrophe theory, but it turned out to be a catastrophe when
applied to human behavior. Perhaps Periera and Doyle have a "calculus"
to offer.
Lacking such a appropriate mathematics, however, does not stop a
theoretical psycholology from existing. In fact, I offer three recent examples
of what a theoretical psychology ought to be doing at this time:
Tversky, A. Features of similarity. PSYCHOLOGICAL REVIEW, 1977, 327-352.
Schank, R.C. DYNAMIC MEMORY. Cambridge University Press, 1982.
Anderson, J.R. THE ARCHITECTURE OF COGNITION. Harvard University Press, 1983.
------------------------------
Date: Thu 29 Sep 83 19:03:40-PDT
From: PEREIRA@SRI-AI.ARPA
Subject: Self-description, multiple levels, etc.
For a brilliant if tentative attack of the questions noted by
Prem Devanbu, see Brian Smith's thesis "Reflection and Semantics
in a Procedural Language,", MIT/LCS/TR-272.
Fernando Pereira
------------------------------
Date: 27 Sep 83 22:25:33-PDT (Tue)
From: pur-ee!uiucdcs!marcel @ Ucb-Vax
Subject: reflexive reasoning ? - (nf)
Article-I.D.: uiucdcs.3004
I believe the pursuit of "consciousness" to be complicated by the difficulty
of defining what we mean by it (to state the obvious). I prefer to think in
less "spiritual" terms, say starting with the ability of the human memory to
retain impressions for varying periods of time. For example, students cramming
for an exam can remember long lists of things for a couple of hours -- just
long enough -- and forget them by the end of the same day. Some thoughts are
almost instantaneously lost, others last a lifetime.
Here's my suggestion: let's start thinking in terms of self-observation, i.e.
the construction of models to explain the traces that are left behind by things
we have already thought (and felt?). These models will be models of what goes
on in the thought processes, can be incorrect and incomplete (like any other
model), and even reflexive (the thoughts dedicated to this analysis leave
their own traces, and are therefore subject to modelling, creating notions
of self-awareness).
To give a concrete (if standard) example: it's quite reasonable for someone
to say to us, "I didn't know that." Or again, "Oh, I just said it, what was
his name again ... How can I be so forgetful!"
This leads us into an interesting "problem": the fading of human memory with
time. I would not be surprized if this was actually desirable, and had to be
emulated by computer. After all, if you're going to retain all those traces
of where a thought process has gone; traces of the analysis of those traces,
etc; then memory would fill up very quickly.
I have been thinking in this direction for some time now, and am working on
a programming language which operates on several of the principles stated
above. At present the language is capable of responding dynamically to any
changes in problem state produced by other parts of the program, and rules
can even respond to changes induced by themselves. Well, that's the start;
the process of model construction seems to me to be by far the harder part
of the task.
It becomes especially interesting when you think about modelling what look
like "levels" of self-awareness, but could actually be manifestations of just
one mechanism: traces of some work, which are analyzed, thus leaving traces
of self-analysis; which are analyzed ... How are we to decide that the traces
being analyzed are somehow different from the traces of the analysis? Even
"self-awareness" (as opposed to full-blown "consciousness") will be difficult
to understand. However, at this point I am convinced that we are not dealing
with a potential for infinite regress, but with a fairly simple mechanism
whose results are hard to interpret. If I am right, we may have some thinking
to do about subject-object distinctions.
In case you're interested in my programming language, look for some papers due
to appear shortly:
Logic-Programming Production Systems with METALOG. Software Practice
and Experience, to appear shortly.
METALOG: a Language for Knowledge Representation and Manipulation.
Conf on AI (April '83).
Of course, I don't say that I'm thinking about "self-awareness" as a long-term
goal (my co-author isn't) ! If/when such a goal becomes acceptable to the AI
community it will probably be called something else. Doesn't "reflexive
reasoning" sound more scientific?.
Marcel Schoppers,
Dept of Comp Sci,
U of Illinois @ Urbana-Champaign
uiucdcs!marcel
------------------------------
Date: 27 Sep 83 19:24:19-PDT (Tue)
From: decvax!genrad!security!linus!philabs!cmcl2!floyd!vax135!ariel!ho
u5f!hou5e!hou5d!mat@Ucb-Vax
Subject: Re: the Halting problem.
Article-I.D.: hou5d.674
I may be naive, but it seems to me that any attempt to produce a system that
will exhibit conciousness-;like behaviour will require emotions and the
underlying base that they need and supply. Reasoning did not evolve
independently of emotions; human reason does not, in my opinion, exist
independently of them.
Any comments? I don't recall seeing this topic discussed. Has it been? If
not, is it about time to kick it around?
Mark Terribile
hou5d!mat
------------------------------
Date: 28 Sep 83 12:44:39-PDT (Wed)
From: ihnp4!drux3!drufl!samir @ Ucb-Vax
Subject: Re: the Halting problem.
Article-I.D.: drufl.674
I agree with mark. An interesting book to read regarding conciousness is
"The origin of conciousness in the breakdown of bicamaral mind" by
Julian Jaynes. Although I may not agree fully with his thesis, it did
get me thinking and questioning about the usual ideas regarding
conciousness.
An analogy regarding conciousness, "emotions are like the roots of a
plant, while conciousness is the fruit".
Samir Shah
AT&T Information Systems, Denver.
drufl!samir
------------------------------
Date: 30 Sep 83 13:42:32 EDT
From: BIESEL@RUTGERS.ARPA
Subject: Recursion of reperesentations.
Some of the more recent messages have questioned the possibility of
producing programs which can "understand" and "create" human discourse,
because this kind of "understanding" seems to be based upon an infinite
kind of recursion. Stated very simply, the question is "how can the human
mind understand itself, given that it is finite in capacity?", which
implies that humans cannot create a machine equivalent of a human mind,
since (one assumes) that underatnding is required before construction
becomes possible.
There are two rather simple objections to this notion:
1) Humans create minds every day, without understanding
anything about it. Just some automatic biochemical
machinery, some time, and exposure to other minds
does the trick for human infants.
2) John von Neumann, and more recently E.F. Codd
demostrated in a very general way the existence
of universal constructors in cellular automata.
These are configurations in cellular space which
able to construct any configuration, including
copies of themselves, in finite time (for finite
configurations)
No infinite recursion is involved in either case, nor is "full"
understanding required.
I suspect that at some point in the game we will have learned enough about
what works (in a primarily empirical sense) to produce machine intelligence.
In the process we will no doubt learn a lot about mind in general, and our
own minds in particular, but we will still not have a complete understanding
of either.
Peolpe will continue to produce AI programs; they will gradually get better
at various tasks; others will combine various approaches and/or programs to
create systems that play chess and can talk about the geography of South
America; occasionally someone will come up with an insight and a better way
to solve a sub-problem ("subjunctive reference shift in frame-demon
instantiation shown to be optimal for linearization of semantic analysis
of noun phrases" IJCAI 1993); lay persons will come to take machine intelligence
for granted; AI people will keep searching for a better definition of
intelligence; nobody will really believe that machines have that indefinable
something (call it soul, or whatever) that is essential for a "real" mind.
Pete Biesel@Rutgers.arpa
------------------------------
Date: 29 Sep 83 14:14:29 EDT
From: SOO@RUTGERS.ARPA
Subject: Top-Down? Bottom-Up?
[Reprinted from the Rutgers bboard.]
I happen to read a paper by Michael A. Arbib about brain theory.
The first section of which is "Brain Theory: 'Bottom-up' and
'Top-Down'" which I think will shed some light on our issue of
top-down and bottom-up approach in machine learning seminar.
I would like to quote several remarks from the brain theorist
view point to share with those interesed:
" I want to suggest that brain theory should confront the 'bottom-up'
analyses of neural modellling no only with biological control theory but
also with the 'top-down' analyses of artificial intelligence and congnitive
psychology. In bottom-up analyses, we take components of known function, and
explore ways of putting them together to synthesize more and more complex
systems. In top-down analyses, we start from some complex functional behavior
that interests us, and try to determine what are natural subsystems into which
we can decompose a system that performs in the specified way. I would argue
that progress in brain theory will depend on the cyclic interaction of these
two methodologies. ..."
" The top-down approach complement bottom-up studies, for one cannot simply
wait until one knows all the neurons are and how they are connected to then
simulate the complete system. ..."
I believe that the similar philosophy applies to the machine learning study
too.
For those interested, the paper can be found in COINS techical report 81-31
by M. A. Arbib "A View of Brain Theory"
Von-Wun,
------------------------------
Date: Fri, 30 Sep 83 14:45:55 PDT
From: Rik Verstraete <rik@UCLA-CS>
Subject: Parallelism and Physiology
I would like to comment on your message that was printed in AIList Digest
V1#63, and I hope you don't mind if I send a copy to the discussion list
"self-organization" as well.
Date: 23 Sep 1983 0043-PDT
From: FC01@USC-ECL
Subject: Parallelism
I thought I might point out that virtually no machine built in the
last 20 years is actually lacking in parallelism. In reality, just as
the brain has many neurons firing at any given time, computers have
many transistors switching at any given time. Just as the cerebellum
is able to maintain balance without the higher brain functions in the
cerebrum explicitly controlling the IO, most current computers have IO
controllers capable of handling IO while the CPU does other things.
The issue here is granularity, as discussed in general terms by E. Harth
("On the Spontaneous Emergence of Neuronal Schemata," pp. 286-294 in
"Competition and Cooperation in Neural Nets," S. Amari and M.A. Arbib
(eds), Springer-Verlag, 1982, Lecture Notes in Biomathematics # 45). I
certainly recommend his paper. I quote:
One distinguishing characteristic of the nervous system is
thus the virtually continuous range of scales of tightly
intermeshed mechanisms reaching from the macroscopic to the
molecular level and beyond. There are no meaningless gaps
of just matter.
I think Harth has a point, and applying his ideas to the issue of parallel
versus sequential clarifies some aspects.
The human brain seems to be parallel at ALL levels. Not only is a large
number of neurons firing at the same time, but also groups of neurons,
groups of groups of neurons, etc. are active in parallel at any time. The
whole neural network is a totally parallel structure, at all levels.
You pointed out (correctly) that in modern electronic computers a large
number of gates are "working" in parallel on a tiny piece of the problem,
and that also I/O and CPU run in parallel (some systems even have more than
one CPU). However, the CPU itself is a finite state machine, meaning it
operates as a time-sequence of small steps. This level is inherently
sequential. It therefore looks like there's a discontinuity between the
gate level and the CPU/IO level.
I would even extend this idea to machine learning, although I'm largely
speculating now. I have the impression that brains not only WORK in
parallel at all levels of granularity, but also LEARN in that way. Some
computers have implemented a form of learning, but it is almost exclusively
at a very high level (most current AI on learning work is at this level),
or only at a very low level (cf. Perceptron). A spectrum of adaptation is
needed.
Maybe the distinction between the words learning and self-organization is
only a matter of granularity too. (??)
Just as people have faster short term memory than long term memory but
less of it, computers have faster short term memory than long term
memory and use less of it. These are all results of cost/benefit
tradeoffs for each implementation, just as I presume our brains and
bodies are.
I'm sure most people will agree that brains do not have separate memory
neurons and processing neurons or modules (or even groups of neurons).
Memory and processing is completely integrated in a human brain.
Certainly, there are not physically two types of memories, LTM and STM.
The concept of LTM/STM is only a paradigm (no doubt a very useful one), but
when it comes to implementing the concept, there is a large discrepancy
between brains and machines.
Don't be so fast to think that real computer designers are
ignorant of physiology.
Indeed, a lot of people I know in Computer Science do have some idea of
physiology. (I am a CS major with some background in neurophysiology.)
Furthermore, much of the early CS emerged from neurophysiology, and was an
explicit attempt to build artificial brains (at a hardware/gate level).
However, although "real computer designers" may not be ignorant of
physiology, it doesn't mean that they actually manage to implement all the
concepts they know. We still have a long way to go before we have
artificial brains...
The trend towards parallelism now is more like
the human social system of having a company work on a problem. Many
brains, each talking to each other when they have questions or
results, each working on different aspects of a problem. Some people
have breakdowns, but the organization keeps going. Eventually it comes
up with a product, although it may not really solve the problem posed
at the beginning, it may have solved a related problem or found a
better problem to solve.
Again, working in parallel at this level doesn't mean everything is
parallel.
Another copyrighted excerpt from my not yet finished book on
computer engineering modified for the network bboards, I am ever
yours,
Fred
All comments welcome.
Rik Verstraete <rik@UCLA-CS>
PS: It may sound like I am convinced that parallelism is the only way to
go. Parallelism is indeed very important, but still, I believe sequential
processing plays an important role too, even in brains. But that's a
different issue...
------------------------------
End of AIList Digest
********************