Copy Link
Add to Bookmark
Report
AIList Digest Volume 5 Issue 044
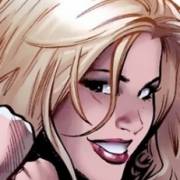
AIList Digest Saturday, 14 Feb 1987 Volume 5 : Issue 44
Today's Topics:
AI Methodology - Symbolic Logic vs. Analog Representation &
Pragmatic Definitions of AI/Cognitive Terms
----------------------------------------------------------------------
Date: 12 Feb 87 17:52:55 GMT
From: vax1!czhj@cu-arpa.cs.cornell.edu (Ted )
Subject: Re: Learing about AI
In article <12992@sun.uucp> lyang%jennifer@Sun.COM (Larry Yang) writes:
>....
>I was in for a surprise. Based on my experience, if you want
>to learn about hard-core, theoretical artificial intelligence,
>then you must have a strong (I mean STRONG) background in formal
>logic.
This is EXACTLY the problem with AI research as it is commonly done today.
(and perhaps yesterday as well). The problem is that mathematicians, logicians
and computer scientists, with their background in formal logic have no other
recourse than to attack the AI problem using these tools that are available
to them. Perhaps this is why the field makes such slow progress?
AI is an ENORMOUS problem, to say the least and research into it should not
be bound by the conventional thinking that is going on. We have to look at
the problem in NEW ways in order to make progress. I am strongly under the
impression that people with a strict theoretical training will actually HINDER
the field rather than advance it because of the constraints on the ideas that
they come up with just because of their background.
Now, I'm NOT saying that nobody in CS, MATH, or LOGIC is capable of original
thought, however, from much of the research that is being done, and from the
scope of the discussions on the NET, it seems safe to say that many people of
these disciplines discount less formal accounts as frivolous.
But look at the approach that LOGIC gives AI. It is a purely reductionist
view, akin to studying global plate motion at the level of sub-atomic
particles. It is simply the wrong level at which to approach the problem.
A far more RATIONAL approach would be to integrate a number of disciplines
towards the goal of understanding intelligence. COMPUTER SCIENCE has a major
role because of the power of computer modeling, efficient data structures and
models of efficient parallel computation. Beyond that, it seems that
computer science should take a back seat. LOGIC, well, where would that fit
in? Maybe at the very lowest level, but most of that is taken for granted by
computer science. PHILOSOPHY tends to be a DEAD END, as can clearly be noted
by the arguments going on on the NET :) Honestly, the philosophy arguments
tend to get so jumbled (though logical), that they really add little to the
field. COGNITIVE PSYCHOLOGY is a quickly emerging field that is producing some
interesting findings, however, at this stage, it is more descriptive than
anything else. There is some interesting speculation into the processes that
are going on behind thought in this field, and they should be looked at
carefully. However, there is simply so much fluff and pointless experiments
that it takes quite a while to wade through and get anything significant.
LINGUISTICS is a similar field. The work of Chomsky and others has given us
some fascinating ideas and may get somewhere in terms of biological constraints
on knowledge and such. Even NEUROBIOLOGY should get involved. Reasearch in
this field gives us more insight into internal constraints. Furthermore,
by studying people with brain disorders (both congenital and through accident)
we can gain some insight into what types of structures are innate or have a
SPECIFIC locus of control.
In sum, I call for using many different disciplines to solve the basic problems
in knowledge, learning and perception. No single approach will do.
---Ted Inoue
------------------------------
Date: 13 Feb 87 14:17:24 GMT
From: sher@CS.ROCHESTER.EDU (David Sher)
Subject: Re: Learing about AI
If I didn't respond to this I'd have to work on my thesis so here
goes:
I think there seems to be something of a misconception regarding the
place of logic wrt AI and computer science in general. To start with
I will declare this:
Logic is a language for expressing mathematical constructs.
It is not a science and as far as artificial intelligence is concerned
the mathematics of logic are not very relevant. Its main feature
is that it can be used for precise expression.
So why use logic rather than a more familiar language, like english.
One can be precise in english, writers like Edgar Allen Poe, Issac
Asimov, and George Gamov all have written very precise english on a
variety of topics. However the problem is that few of us knowledge
engineers have the talent to be precise in our everyday language.
There are few great, or even very good writers among AI practitioners.
Thus for decades engineers, scientists, and statisticians have used
logic to express their ideas since even an incompetent speaker can be
clear and precise using logical formalisms. However like any language
with expressive power one can be totally incomprehensible using logic.
I have seen logical expressions that even the author did not
understand. Thus logic is not a panacea, it is merely a tool. But it
is a very useful and important tool (you can chop down trees with a
boy scout knife but I'll take an axe any day and a chain saw is even
better). Also like english or any other language the more logic you
know the more clearly and compactly you can state your ideas (if you
can avoid the temptation to use false erudition and use your document
to demonstrate your formal facility rather than what you are trying to
say). Thus if you know modal or second order logics you can express
more than you can with simple 1st order predicate calculus and you can
express it better.
Of course, not everyones goals are to express themselves clearly.
Some people's business is to confuse and obfuscate. While logic can
be put to this purpose it is easier to use english for this task. It
takes an uncommon level of expertise to be really confusing without
appearing incompetant with logic.
Note: I am not a logician but I use a lot of logic in my everyday
work which is probabilistic analysis of computer vision problems
(anyone got a job?).
--
-David Sher
sher@rochester
{allegra,seismo}!rochester!sher
------------------------------
Date: Fri, 13 Feb 87 14:02:51 pst
From: Ray Allis <ray@BOEING.COM>
Subject: Other Minds
Some of you may be after the fame and great wealth associated with AI
research, but MY goal all along has been to BUILD an "other mind"; a
machine who thinks *at least* as well as I do. If current "expert
systems" are good enough for you, please skip this. Homo Sap.'s
distinguished success among inhabitants of this planet is primarily due
to our ability to think. We will continue to exist only if we act
intelligently, and we can use all the help we can get. I am not
convinced that Mutual Assured Destruction is the most intelligent
behavior we can come up with. It's clear the planetary population can
benefit from help in the management of complexity, and it is difficult
for me to imagine a goal more relevant than improving the chances for
survival by increasing our ability to act intelligently.
However, no machine yet thinks nearly as well as a human, let alone
better. I wouldn't trust any computer I know to babysit my child, or
my country. Why? Machines don't understand! Anything! The reason
for this poor performance is an inadequate paradigm of human intelligence.
The Physical Symbol System Hypothesis does not in fact account for human
intelligent behavior.
Parenthetically, there's no more excitement in symbol-processing computers;
that's what digital computers have been doing right along, taking the
symbol for two and the symbol for two, performing the defined operation
"ADD" and producing the symbol for four. We may have lost interest in
analog systems prematurely.
Manipulation of symbols is insufficient by itself to duplicate human
performance; it is necessary to treat the perceptions and experiences the
symbols *symbolize*. Put a symbol for red and a symbol for blue in a pot,
and stir as you will, there will be no trace of magenta.
I have developed a large suite of ideas concerning symbols and
representations, analog and digital "computing", induction and
deduction, natural language, consciousness and related concepts which
are inextricably intertwined and somewhat radical, and the following
is necessarily a too-brief introduction. But maybe it will supply
some fuel for discussion.
Definition of terms: By intelligence, I mean intelligent behavior;
intelligent is an adjective describing behavior, and intelligence is a name
for the ability of an organism to behave in a way we can call intelligent.
Symbols and representations: There are two quite distinct notions denoted
by *symbolize* and *represent*. Here is an illustration by example:
Voodoo dolls are intended as symbols, not necessarily as faithful images
of a person. A photo of your family is representative, not symbolic. A
picture of Old Glory *represents* a flag, which in turn *symbolizes* some
concepts we have concerning our nation. An evoked potential in the visual
cortex *represents* some event or condition in the environment, but does
not *symbolize* it.
The essence of this notion of symbolism is that humans can associate
phenomena "arbitrarily"; we are not limited to representations. Any
phenomenon can "stand for" any other. That which any symbol symbolizes
is a human experience. Human, because we appear to be the only symbol
users on the planet. Experience, because that is symbolism's ultimate
referent, not other symbols. Sensory experience stops any recursion.
Noises and marks "symbolize" phenomenological experience, independent of
whether those noises and marks are "representative".
Consciousness: Consciousness is self-consciousness; you aren't conscious
of your environment, you are conscious of your perceptions of your
environment. Sensory neurons synapse in the thalamus. From there,
neurons project to the cortex, and from the cortex, other neurons project
back to the thalamus, so there, in associative contiguity, lie the input
lines and reflections of the results of the perceptive mechanisms. The
brain has information as to the effects of its own actions. Whether it is
resident in thalamic neurons or distributed throughout the brain mass, that
loop is where YOU are, and life experience builds your identity; that hand
is part of YOU, that hammer is not. One benefit of consciousness is that
it extends an organism's time horizon into the past and the future,
improving its chance for survival. Consciousness may be necessary for
symbol use.
Natural language: Words, spoken or written, are *symbols*. But human
natural language is not a symbol system; there are no useful interactions
among the symbols themselves. Human language is evocative; its function
is to evoke experiences in minds, including the originating mind. Words
do not interact with each other; their connotations, the evoked responses
in human minds interact with each other. Responses are based on human
experience; touch, smell, vision, sound, emotional effects. Communication
between two minds requires some "common ground"; if we humans are to
communicate with the minds we create, we and they must have some
experiential "common ground". That's why no machine will "really
understand" human natural language until that machine can possess the
experiences the symbols evoke in humans.
Induction and deduction: Induction, as defined here, consists in the
cumulative effect of experience on our behavior, as implemented by neural
structures and components. Induction is the effect on an organism's
behavior; not a procedure effected by the organism. That is to say, the
"act" of induction is only detectable through its effects. All living
organisms' behavior is modified by experience, though only humans seem
to be self-aware of the phenomenon. Induction treats *representations*,
rather than *symbols*; the operation is on *representation* of experience,
quite different from symbolic deduction.
Deduction treats the *relationships among symbols*, that which Hume
described as "Relations of Ideas". There is absolute certainty concerning
all valid operations, and hence the resulting statements. The intent is
to manipulate a specific set of symbols using a specific set of operations
in a mechanical way, having made the process sufficiently explicit that we
can believe in the results. But deduction is an operation on the *form*
of a symbol system; a "formal" operation, and deliberately says nothing at
all concerning the content. Deductive, symbolic reasoning may be the
highest ability of humans, but there's more to minds than that.
Analogy: One definition of analogy is as the belief that if two objects or
events are alike in some observed attributes they are alike in other,
unobserved, attributes. It follows that the prime requisite for analogy
is the perception of "similarity". It could be argued that the detection
of similarity is one of the most basic abilities an organism must have to
survive. Similarity and analogy are relationships among *representations*,
not among *symbols*. Significant similarities, (i.e. analogy and metaphor)
are not to be found among the symbols representing mental perceptions, but
among the perceptions themselves. Similarity is perceived among
experiences, as recorded in the central nervous system. The mechanism is
that symbols evoke, through association, the identical effects in the
nervous system as are evoked by the environmental senses. Associative
memory operates using sensory phenomena; that is, not symbols, but *that
which is symbolized* and evoked by the symbols. We don't perceive
analogies between symbols, but between the experiences the symbols evoke
in our minds.
Analog and digital: The physical substrate supporting intelligent behavior
in humans is the central nervous system. The model for understanding the
CNS is the analog "gadget" which "solves problems", as in A. K. Dewdney's
Scientific American articles, not Von Neumann computers; nor symbol
systems of any kind. The "neural net" approaches look promising, if they
are considered to be modifiable analog devices, rather than alternative
designs for algorithmic digital computers.
Learning and knowledge: Learning is inductive; by definition the addition
of knowledge. "Deductive logic is tautological"; i.e. implications of
present knowledge can be made explicit, but no new knowledge is introduced
by deductive operations. There is no certainty with induction, though:
"And this kind of association is not confined to men; in
animals also it is very strong. A horse which has been
often driven along a certain road resists the attempt to
drive him in a different direction. Domestic animals
expect food when they see the person who usually feeds them.
We know that all these rather crude expectations of
uniformity are liable to be misleading. The man who has
fed the chicken every day throughout its life at last
wrings its neck instead, showing that more refined views
as to the uniformity of nature would have been useful to
the chicken."
[Bertrand Russell. 1912. "On Induction", Problems of Philosophy.]
Thinking systems will be far too complex for us to construct in "mature"
form; artificial minds must learn. Our most reasonable approach is to
specify the initial conditions is terms of the physical implementation
(e.g., sensory equipment and pre-wired associations) and influence the
experience to which a mind is exposed, as with our children.
What is meant by "learning"? One operational definition is this: can you
apply your knowledge in appropriate ways? Some behavior must be modified.
All through your childhood, all through life, your parents and teachers
are checking whether you have learned something by asking you to apply it.
As a generalization of applying, a teacher will ask if you can re-phrase
or restate your knowledge. This demonstrates that you have internalized
it, and can "translate" from internal to external, in symbols or in modified
behavior. Language to internalized, and back to language... if you can
do this, you "understand".
Knowledge is the state of the central nervous system, either built in or
acquired through experience. Experience is recorded in the CNS paths which
"process" it. Recording experience essentially in the same lines which
sense it saves space and totally eliminates access time. There is no
retrieval problem; re-evocation, re-stimulation of the sensory path is
retrieval, and that can be done by association with other experience, or
with symbols.
That's probably enough for one shot. Except to say I think the time
is ripe for trying some of these ideas out on real machines. A few years
ago there was no real possibility of building anything so complex as a
Connection Machine or a million-node "neural net", and there's still no
chance at constructing something as complex as a baby, but maybe there's
enough technology to build something pretty interesting, anyway.
Ray
------------------------------
End of AIList Digest
********************