Copy Link
Add to Bookmark
Report
Machine Learning List Vol. 6 No. 10
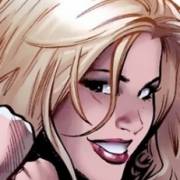
Machine Learning List: Vol. 6 No. 10
Wednesday, March 30, 1994
Contents:
Georgia Tech CogSci/AI WWW pages
MLnet Summer School & Workshop on Machine Learning
MLC '94 Workshop on Robot Learning announcement
AAAI Fall Symposium -- Improving Instruction of Introductory AI
Registration form for ML94/COLT94 (Plain text format)
Second Call for Papers
NEW BOOK: Simply Logical - Intelligent Reasoning by Example
AUTONOMOUS LEARNING FROM THE ENVIRONMENT
The Machine Learning List is moderated. Contributions should be relevant to
the scientific study of machine learning. Mail contributions to ml@ics.uci.edu.
Mail requests to be added or deleted to ml-request@ics.uci.edu. Back issues
may be FTP'd from ics.uci.edu in pub/ml-list/V<X>/<N> or N.Z where X and N are
the volume and number of the issue; ID: anonymous PASSWORD: <your mail address>
----------------------------------------------------------------------
Date: Fri, 25 Mar 1994 14:53:56 -0500
From: Ashwin Ram <ashwin@cc.gatech.edu>
Subject: Georgia Tech CogSci/AI WWW pages
ML folks might be interested in the following home pages for the Cognitive
Science and Artificial Intelligence programs at Georgia Tech in Atlanta,
which contain, among other things, information on machine learning projects
and technical reports which can be downloaded at the click of a button, and a
keyword-searchable index of available papers:
Cognitive Science: http://www.gatech.edu/cogsci/cogsci.html
Artificial Intelligence: http://www.gatech.edu/ai/ai.html
The site contains information about research projects, on-line technical
reports, list of faculty and students, as well as educational programs and
courses in Computer Science, Psychology, Human-Machine Systems, Philosophy,
and English. Also some info relating to this year's cognitive science
conference which will be held at Georgia Tech.
Feel free to browse -- we love visitors!
--
Ashwin Ram <ashwin.ram@cc.gatech.edu>
Assistant Professor, College of Computing
Georgia Institute of Technology, Atlanta, Georgia 30332-0280
http://www.gatech.edu/ai/faculty/ram.html
------------------------------
From: lola@sun5.lri.fr (Dolores Canamero)
Subject: MLnet Summer School & Workshop on Machine Learning
Date: Mon, 28 Mar 1994 16:53:15 GMT
==============================================
MLNET'S SUMMER SCHOOL - FIRST ANNOUNCEMENT
September 5 - 10, 1994
Dourdan (south neighborhood of Paris), France
==============================================
The Summer School is organized by Celine Rouveirol (LRI, France). Its aim
is to provide training in the latest developments in Machine Learning and
Knowledge Acquisition to AI researchers, but also to industrials who are
investigating possible applications of those techniques. The Summer School
is sponsored by CEC and PRC-IA.
PROVISIONAL PROGRAM
Monday, September 5
___________________________
. Morning: Case-Based Reasoning (Agnar Aamodt, Univ. Trondheim, Norway) [3h]
. Afternoon: Learning and Probabilities (Wray Buntine, RIACS/NASA Ames,
Moffet Field, CA, USA) [3h]
Tuesday, September 6
___________________________
. Morning: Learning and Noise (Ivan Bratko, JSI, Ljubljana, Slovenia) [3h]
. Afternoon: Knowledge Acquisition (Bob Wielinga, Univ. Amsterdam, NL) [3h]
Wednesday, September 7
___________________________
. Morning: Integrated Architectures (Lorenza Saitta, Univ. Torino, Italy) [3h]
. Afternoon: Knowledge Revision (Derek Sleeman, Univ. Aberdeen, UK) [2h],
(Stefan Wrobel, GMD, Bonn, Germany) [2h]
Thursday, September 8
___________________________
. Morning: Knowledge Acquisition and Machine Learning (Maarten van
Someren, Univ. Amsterdam, NL) [3h]
. Afternoon: Reinforcement Learning (Leslie Kaebling, Brown Univ., USA) [3h]
Friday, September 9
___________________________
. Morning: Inductive Logic Programming (Steve Muggleton, Univ. Oxford,UK) [3h]
. Afternoon: Inductive Logic Programming (Celine Rouveirol, LRI, Univ.
Paris-Sud, France) [2h], (Francesco Bergadano, Univ. Catania, Italy) [2h]
Saturday, September 10
___________________________
. Morning: Conceptual Clustering (Gilles Bisson, LIFIA, Grenoble, France) [3h]
Invited conferences and demonstrations of software will be organized during
the evenings.
Requests for information and registration forms are to be addressed to
Dolores Canamero, (ML-SS'94), LRI, Bat. 490, Universite Paris-Sud,
F-91405, Orsay Cedex, France (e-mail: mlss94@lri.fr).
Full and partial grants can be accorded to European students and to members
of PRC-IA groups.
============================================
PROVISIONAL PROGRAM OF MLNET'S WORKSHOP ON
INDUSTRIAL APPLICATIONS OF MACHINE LEARNING
FIRST ANNOUNCEMENT
September 2 and 3, 1994
Dourdan (south neighborhood of Paris), France
============================================
Organizer: Yves Kodratoff (LRI & CNRS, Orsay, France)
The workshop will take place in Dourdan (some 50 km south of Paris), the 2
and 3 September 1994.
The registration fee will be FF. 800.
PROVISIONAL PROGRAM
Second of Sept. 1994: Overview presentations
_______________________________________________________
. Ivan Bratko (Univ. Ljubljana) "On the state-of-the-art of industrial
applications of ML"
. Gregory Piatewski-Shapiro (GTE) "DDB: data mining in data bases"
. XXX "Industrial applications of KADS-II" (if not possible, an overview
of the industrial applications of Knowledge Acquisition is planned)
. Attilio Giordana (Univ. Torino) "Applications of ML to robotics"
. Franz Schmalhofer (DFKI) "Unifying KA and ML for applications"
. Vassilis Moustakis (Univ. Crete) "An overview of applications of ML to
medicine"
Third of Sept. 1994
________________________
Special address:
Setsuo Arikawa "Knowledge Acquisition from Protein Data
by Machine learning System BONSAI"
Results of ESPRIT projects:
. Nick Puzey (BAE) "Industrial applications of MLT"
. Pavel Brazdil (Univ. Porto) "Industrial applications of STATLOG"
. Attilio Giordana (Univ. Torino) "The results of BLEARN"
Reports on results:
. Pieter Adriaans "Application of GAs at Syllogics"
. Fabio Malabocchia "ML at CSELT"
. Juergen Hermann "Learning Rules about VLSI-Design"
. Reza Nakaeizadeh "ML at Daimler-Benz"
. Francois Lecouat "CBR at Matra-Space"
Demos will take place during the evenings. Participants are welcome to
follow the Monday and Tuesday courses of the subsequent ML summer school on
Case-Based Reasoning, ML and Statistics, Noise in Data, Knowledge Acquisition.
Note however that they will have to register specifically to this school.
Requests for information and registration forms are to be addressed to
Dolores Canamero, (ML-SS'94), LRI, Bat. 490, Universite Paris-Sud,
F-91405, Orsay Cedex, France (e-mail: mlss94@lri.fr).
------------------------------
Date: Tue, 22 Mar 94 14:39:17 EST
From: Sridhar Mahadevan <mahadeva@guardian.csee.usf.edu>
Subject: MLC '94 Workshop on Robot Learning announcement
_______________________________________
MLC-COLT '94 WORKSHOP ON ROBOT LEARNING
July 10th 1994
Rutgers Univ., New Brunswick, NJ
________________________________________
DESCRIPTION OF WORKSHOP:
________________________
Building a learning robot has been acknowledged as one of the grand
challenges facing artificial intelligence. Robotics is an extremely
challenging domain for machine learning: sensors generate huge noisy
state spaces, actions are often continuous, and training time is very
limited. The area of robot learning has witnessed a surge of activity
recently, fueled by exciting new work in the areas of reinforcement
learning, behavior-based robotics, genetic algorithms, neural
networks, and artificial life. The goal of this workshop is to
discuss/communicate research ideas from these and other relevant
fields on building learning robots.
This workshop will focus on tasks and learning methods appropriate for
real robots. Many techniques look promising on simulations, but fail
to work on real robots because they take too long, or require
information that cannot be computed reliably from sensors. This
workshop will also discuss a common format for making training data
available across the Internet to enable researchers without access to
real hardware to contribute their ideas and expertise to robot
learning.
We encourage researchers from several different research areas,
including robotics, machine learning, planning, vision, and neural
nets to attend this workshop. This diversity will facilitate
interaction among disparate research groups who face similar problems.
Researchers with knowledge of real robots are especially encouraged to
attend to share their experience.
TOPICS:
_______
The workshop will include (but is not limited to) the following
topics:
1. ADAPTIVE CONTROL ARCHITECTURES: This includes work on
learning new behaviors, learning to coordinate existing behaviors,
and learning in hybrid declarative-reactive architectures.
2. PERCEPTUAL LEARNING: This includes building categories
from sensor data, such as learning to recognize visual objects, or
clustering sonar data.
3. LEARNING SPATIAL MAPS: This includes work in the area of
robot navigation.
4. LEARNING ARM CONTROL AND MANIPULATION: This includes
learning to control robot arms, and learning to pick up objects.
5. LEARNING WITH DOMAIN-SPECIFIC BIAS: Many learning researchers have
come to the conclusion that tabula rasa learning is not effective for
robots. If that is so, we must add domain-specific bias in some form.
What should it be? Examples include teaching, pre-specified
behavioral structure, and pre-specified low-level behaviors.
6. COMPARATIVE STUDIES: Lastly, the workshop will also
encourage comparative studies among different learning methods,
particularly those done using real robots.
FORMAT OF THE WORKSHOP:
_______________________
The workshop will begin with an overview of the current state of the
field, followed by short presentations of current research. There may
be one or two invited talks if time permits. There will be a video
session featuring demos of learning robots. Finally, there will be a
panel discussion to summarize what we've learned and discuss issues
for future research. We plan on also getting together for a workshop
dinner after the workshop.
CALL FOR PAPERS:
________________
Researchers interested in presenting their work are encouraged to
submit papers to the workshop. Please follow the same formatting
guidelines used in the regular ML conference (i.e. 12 pages in Latex
12 pt article style excluding title page and bibliography, but
including all tables and figures). Four copies of each paper should be
mailed to the Chair (see address below). The deadline for receiving
papers is May 1. Authors of accepted papers will be notified by May
21st, and camera-ready papers should be submitted by June 15th. We
will try to distribute the workshop proceedings before the workshop.
CALL FOR VIDEOS:
________________
We also encourage researchers to submit short videos featuring their
robots. We will accept all videos if they are reasonably short (no
more than 10 minutes). Depending on the number of submitted videos,
and the time constraints for the overall length of the video session,
further editing may be required.
ATTENDANCE:
___________
Attendance in this workshop will be by invitation only. All
researchers interested in attending this workshop should submit a one
page abstract outlining their current and previous work in robot
learning. In order to maximize the degree of interaction among the
participants, we will give priority to authors of accepted papers,
invited speakers and panelists, and researchers who have had
experience in working with real robots.
PROGRAM COMMITTEE:
__________________
Leslie Kaelbling Sridhar Mahadevan (Chair)
Box 1910 Dept of Computer Science and Engineering
Dept. of Computer Science University of South Florida
Brown University Tampa, FL 33647
Providence, RI 02192 mahadeva@csee.usf.edu
lpk@cs.brown.edu
Maja Mataric Tom Mitchell
MIT AI Lab School of Computer Science
Cambridge, MA 02139 Carnegie-Mellon University
maja@ai.mit.edu Pittsburgh, PA 15213
mitchell@cs.cmu.edu
------------------------------
From: Marti Hearst <marti@cs.berkeley.edu>
Subject: AAAI Fall Symposium -- Improving Instruction of Introductory AI
Call for Participation
Improving Instruction of Introductory
Artificial Intelligence (IIIA)
To be held as part of the:
AAAI 1994 Fall Symposium Series
November 4-6, 1994
New Orleans, Louisiana
Introductory Artificial Intelligence is a notoriously difficult course
to teach well. The two most straightforward strategies are to either
present a smorgasbord of topics or to focus on one or two central
approaches. The first option often yields disjointed and superficial
results, whereas the second presents an incorrectly biased picture.
Often students come away with a feeling that the subject matter is not
coherent and that the field has few significant achievements.
Other issues instructors must face are: What should the balance be between
the emphasis on cognitive modeling vs. engineering solutions to hard
problems? How should one handle the well-known phenomenon of
solutions that work no longer being considered to be part of AI?
What strategies do instructors take to deal with these issues? What are some
underlying themes that can be used to help structure the material? Are there
sets of principles that can be used to instruct the material, even if they
do not precisely reflect all of the current viewpoints?
We propose that the AI community meet and tackle these issues together.
The goal of the symposium is to provide an opportunity to discuss these
difficult questions, as well as to share successful strategies, problem
assignments, instructional programs, and instructional "bloopers."
The symposium will be organized as a workshop, although there will be
tutorial aspects, allowing participants to learn from the experiences of
colleagues who have worked out successful solutions. All attendees will
participate in less formal breakout sessions as well as in discussion
following presentations.
We are soliciting four kinds of contributions, corresponding to four
presentation types.
Type I
A discussion of a successful strategy for instructing introductory AI, based
on experience. The descriptions should be centered around a syllabus and
must describe how the strategy handles the smorgasbord versus bias
problem, i.e., what underlying unifying themes give cohesiveness to the
approach while at the same time covering the material well. Accepted
descriptions (one to three pages plus the syllabus) will appear in the
working notes, and the authors of some of these will be asked to present
these ideas in the form of a talk.
Those submitting papers on successful overall approaches should also
address the following issues, and optionally those listed under Type II as
well.
1) What are the basics that must be covered, and how are they integrated
into the theme of the course?
2) One strategy is to describe a problem to be solved and then discuss
several different techniques that attempt to solve the problem. The inverse
strategy describes a technique and then explores how well it performs on
different problem types. Which, if either, strategy is used?
3) What kind of curriculum does this fit in to? (E.g., isolated undergraduate
semester, part of an intelligent systems series, overview for graduate
students, etc.)
Type II
A position paper addressing at least one of the following issues (one to four
pages). Selected position papers will appear in the working notes and some
authors will be asked to participate in well-structured, strongly moderated
panel discussions (i.e., answers to a small set of questions prepared in
advance).
Content issues include:
1) What is the role of cognitive motivation, if any? Should there be an
emphasis on simulating intelligence, modeling the mind, etc.?
2) What is the role of formalism?
3) Should commercially feasible aspects be addressed, and if so, how?
4) How should we address the question, is it still AI if it can be done?
5) What role should advanced areas (e.g., vision, robotics) play?
6) What kind of hooks should be left for more in-depth courses (e.g.,
graduate ML, NLP, vision, KR, connectionism, etc.)?
7) What is the role of historical developments? Should this be a structuring
theme?
Curricular issues include:
1) What kind of department; interaction with other subareas, e.g.: cognitive
systems, intelligent systems.
2) What kind of educational program: One semester or quarter overview? A
two or three semester series centered around a theme (e.g., KR)?
3) Undergraduate versus graduate: How much should they overlap?
Remedial introduction for those who never took undergraduate AI? What
are the positive and negative results? Undergrad pedagogical, hands on
experience? Graduate reading: primary sources?
Teaching college perspective issues include:
1) What are the special issues?
2) How can pooled resources help?
Laboratory involvement issues include:
1) Is programming useful or a time waster? Is it better to use and observe
existing tools instead? Or is a compromise--modifying existing programs--
best?
Innovative ideas?
Type III
Informative descriptions of programming tools for assignments and as
instructional aids. Many potential symposium participants have expressed
interest in acquiring a collection of useful programs both for demonstration
of AI concepts and for use in homework assignments in lieu of requiring
students to spend their time programming. This is an opportunity to
advertise tools, videotapes, and other teaching aids. We would like all
participants that have used tools that they have found to be useful to list
these tools, and all developers of new tools to describe them. For those who
have created new tools, we would like to create a repository of same.
Type III submissions should address at least one of the following (one to
five pages):
1) Descriptions of new educational tools specifically designed for
instruction of AI (e.g., The FLAIR project of Papalaskari et al.).
2) Recommendations and pans of existing tools as instructional aids.
3) Proposals for tools or tool construction methodologies that don't exist
but should.
4) Tools for empirical exploration (e.g., a tutorial guide to the tools in the
machine learning repository, as applied for instructional purposes).
Type IV
Contributory questions (one to two pages). Those wishing to participate but
who do not wish to contribute in one of the previous categories must
indicate their interest by describing what issues they are interested in
(including those listed above) and suggesting questions to be addressed by
the symposium. Descriptions of bloopers--mistakes made that others
should be warned away from, are also appropriate. We are especially
interested in topics to be discussed by small groups, and questions to be
asked of the panelists.
Submission Information:
Authors may send contributions of more than one type, if desired. Only one
document should be submitted. A submitter who wants to make more than
one type of submission should simply label each part of the document with
the appropriate type (Type I, Type II, etc).
Email submissions are strongly preferred. Send one copy, plain text, to:
marti@cs.berkeley.edu. PostScript is acceptable if it is accompanied by a
plain text version as well; this way diagrams can be included in an electronic
form. Those people who cannot send an electronic version should send
three hard copies to:
Marti Hearst
Chair, Symposium on Improving Instruction of Introductory AI
Xerox PARC
3333 Coyote Hill Rd
Palo Alto, CA 94304
Organizing Committee
Marti Hearst (chair), UC Berkeley; Haym Hirsh, Rutgers; Dan
Huttenlocher, Cornell; Nils Nilsson, Stanford; Bonnie Webber, University
of Pennsylvania; Patrick Winston, MIT.
===========================
Sponsored by the
American Association for Artificial Intelligence
445 Burgess Drive, Menlo Park, CA 94025
(415) 328-3123
fss@aaai.org
Symposia will be limited to between forty and sixty participants. Each
participant will be expected to attend a single symposium. Working notes
will be prepared and distributed to participants in each symposium.
[NB: the IIIA symposium may be made larger if there is a perceived
need. --MH]
A general plenary session, in which the highlights of each symposium will
be presented, will be held on November 5, and an informal reception will be
held on November 4.
In addition to invited participants, a limited number of other interested
parties will be able to register in each symposium on a first-come, first-
served basis. Registration will be available by mid-July 1994. To obtain
registration information write to the AAAI at 445 Burgess Drive, Menlo
Park, CA 94025 (fss@aaai.org).
Submission Dates
+ Submissions for the symposia are due on April 15, 1994.
+ Notification of acceptance will be given by May 17, 1994.
+ Material to be included in the working notes of the symposium must be
received by August 19, 1994.
Partial support for travel expenses will be available to some
participants on a need-driven basis.
------------------------------
Date: Tue, 22 Mar 94 14:20 EST
From: William Cohen <wcohen@research.att.com>
Subject: Registration form for ML94/COLT94 (Plain text format)
Below is a plain text version of the (preliminary) registration
information for the ML94 and COLT94 conferences. The message includes
a description of the tutorials and workshops, and also a list of the
technical papers accepted to ML. (Authors: this is probably your
first chance to find out about the decision on your paper. Reviews
are currently on their way via physical mail.) A list of papers
accepted to COLT and the final schedule for technical papers will be
distributed in mid-April, after the COLT program committee finishes
its deliberations.
An unabbreviated version of this announcement in Latex or postscript
can be obtained via anonymous ftp from cs.rutgers.edu in the directory
pub/learning94. If you do not have access to ftp, send email to
ml94@cs.rutgers.edu or colt94@research.att.com.
William Cohen
ML94 Co-Chair
*=*=*=*=*=*=*=*=*=*=*=*=*=*=*=*=*=*=*=*=*=*=*=*=*=*=*=*=*=*=*=*=*=*=*=*=*
--- Preliminary Announcement ---
ML '94 COLT '94
Eleventh International Conference Seventh ACM Conference on
on Machine Learning Computational Learning Theory
July 10-13, 1994 July 12-15, 1994
Rutgers, The State University of New Jersey, New Brunswick
*-*-*-*-*-*-*-*-*-*-*-*-*-*-*-*-*-*-*-*-*-*-*-*-*-*-*-*-*-*-*-*-*-*-*-*-*
The COLT and ML conferences will be held together this year at Rutgers
University in New Brunswick. This is the first time that COLT and ML
will be held in the same location, and we are looking forward to a
lively and interdisciplinary meeting of the two communities. Please
come and help make this exciting experiment a success.
Among the highlights of the conferences are three invited lectures,
and, on Sunday, July 10, a day of workshops and tutorials on a variety
of topics relevant to machine learning. The tutorials are sponsored
by DIMACS, and are free and open to the general public. COLT is
sponsored by the ACM Special Interest Groups on Algorithms and
Computation Theory (SIGACT) and on Artificial Intelligence (SIGART).
In addition, COLT and ML received generous support this year from
AT&T Bell Laboratories and the NEC Research Institute.
This preliminary announcement, which omits the final technical
program, is being provided so that travel arrangements can be made as
early as possible. An updated announcement, including the technical
program, will be distributed sometime in April.
>>>> WARNING <<<< The dates of the conferences coincide this year
with the World Cup soccer matches being held at Giants Stadium in East
Rutherford, New Jersey. These games are expected to be the largest
sporting event ever held in the New York metropolitan area, and it is
possible that the volume of soccer fans in the area could adversely
affect your ability to make travel reservations. Therefore, IT IS
EXTREMELY IMPORTANT THAT YOU MAKE ALL YOUR TRAVEL ARRANGEMENTS AS
EARLY AS POSSIBLE.
GENERAL INFORMATION
LOCATION. The conferences will be held at the College Avenue Campus
of Rutgers University in downtown New Brunswick, which is easily
accessible by air, train, and car. For air travel, New Brunswick is
35 minutes from Newark International Airport, a major U.S. and
international airline hub. By rail, the New Brunswick train station
is located less than four blocks from the conference site and is on
Amtrak's Northeast corridor. For travel by car, the conference site
is approximately three miles from Exit 9 of the New Jersey Turnpike.
See instructions below for obtaining a map of the campus. Most
conference activities will take place in Scott Hall (#21 on map) and
Murray Hall (#22). Conference check-in and on-site registration will
take place in Scott Hall (follow signs for exact room location) on
Saturday, July 9 at 3-6pm, and everday after that beginning at 8am.
REGISTRATION. Please complete the attached registration form, and
return it with a check or money order for the full amount. The early
registration (postmark) deadline is May 27, 1994.
HOUSING. We have established the group rate of $91/night for a single
or a double at the HYATT REGENCY HOTEL (about five blocks from the
conference site). This rate is only guaranteed through June 10, 1994,
and, due to limited availability, it is strongly recommended that you
make reservations as soon as possible. To reserve a room, please call
the Hyatt directly at 908-873-1234 or 800-233-1234 and be sure to
reference ML94 or COLT94. Parking is available at the hotel for a
discounted $3/night.
We have also reserved dormitory space in two dorms, both of which are
an easy walk to the main conference site. Dorm reservations must be
made by the early registration deadline of May 27, 1994. Both dorms
include daily maid service (linens provided first day for the week and
daily fresh towels and beds made). The Stonier Hall dorms (#56 on
map) are air-conditioned with private bath and are situated in the
center of the campus. Due to limited availability, only shared double
rooms are available in Stonier. Only a block away, the Campbell Hall
dorms (#50) are one of a set of three "river dorms" overlooking the
Raritan River. Although Campbell Hall is not air-conditioned, the
view of the river is quite pleasing and rooms on the river side should
offer good air flow. Baths in Campbell are shared on each floor, with
single and double rooms available.
Please specify your dorm preference on your registration form, and we
will assign space accordingly on a first come, first served basis as
long as rooms are available. Unfortunately, because there are only a
finite number of rooms within each dormitory, we cannot absolutely
guarantee your request. Check-in for the dorms will take place at the
Housing Office in Clothier Hall (#35) which is located next to the
Hurtado Health Center (#37) on Bishop Place. Check-in hours will be
4pm to midnight, July 9-13. Parking passes, for those staying in the
dorms, will be available upon check-in.
TRAVEL BY AIR. Newark International Airport is by far the most
convenient. A taxi from the airport to New Brunswick costs about $36
(plus nominal tolls) for up to four passengers. (This is the
flat-rate fare for a _licensed_ taxi from the official-looking taxi
stand; it is strongly recommended that you refuse rides offered by
unlicensed taxi drivers who may approach you elsewhere in the
airport.) Shuttle service to New Brunswick is available from ICS for
$23 per person. ICS shuttles run direct to the Hyatt, and require at
least one-day advance reservations (908-566-0795 or 800-225-4427). If
renting a car, follow signs out of the airport to New Jersey Turnpike
South, and continue with the directions below. By public
transportation, take the Airlink bus ($4 exact fare) to Newark Penn
Station and follow the "by rail" directions below. (New Jersey
Transit train fare is $5.25 one-way or $8 round trip excursion; trains
run about twice an hour during the week, and less often in the evening
and on weekends.)
TRAVEL BY CAR. Take the New Jersey Turnpike (south from Newark or New
York, north from Philadelphia) to Exit 9. Follow signs onto Route 18
North or West (labeled differently at different spots) toward New
Brunswick. Take the Route 27, Princeton exit onto Albany Street
(Route 27) into downtown New Brunswick. The Hyatt Regency Hotel will
be on your left after the first light. If staying at the Hyatt, turn
left at the next light, Neilson Street, and left again into the front
entrance of the hotel. If staying in the dorms, continue past this
light to the following light, George Street, and turn right. Stay on
George Street to just before the fifth street and turn left into the
Parking Deck (#55 on map). Walk to the Housing Office in Clothier
Hall (#35) for dormitory check-in.
TRAVEL BY RAIL. Take either an Amtrak or a New Jersey Transit train
to the New Brunswick train station. This is located at the corner of
Albany Street and Easton Avenue. If staying at the Hyatt Regency
Hotel, it is a (long) three block walk to the left on Albany Street to
the hotel. If staying in the dorms it is a (long) six block walk to
the Housing Office in Clothier Hall (#35 on map) for dormitory
check-in. (The taxi stand is in front of the train station on Albany
Street.)
MEALS. Continental breakfast is included with registration, but not
lunch or dinner. Restaurants abound within walking distance of the
conference and housing venue, ranging from inexpensive food geared to
college students to more expensive dining.
A reception on July 12 is scheduled at the rustic Log Cabin, situated
next to the experimental gardens of the agricultural campus, as part
of the registration package for all ML94 and COLT94 attendees. The
banquet on July 13 is included in the registration package for
everyone except students.
CLIMATE. New Jersey in July is typically hot, with average daily
highs around 85 degrees, and overnight lows around 70. Most days in
July are sunny, but also come prepared for the possibility of
occasional rain.
THINGS TO DO. The newly opened Liberty Science Center is a fun,
hands-on science museum located in Liberty State Park, about 30-45
minutes from New Brunswick (201-200-1000). From Liberty State Park,
one can also take a ferry to the Statue of Liberty and the Immigration
Museum at Ellis Island.
New York City can be reached in under an hour by rail on New Jersey
Transit. Trains run about twice an hour during the week, and once an
hour on weekends and at night. Fare is $7.75 one-way, $11.50 round
trip excursion.
New Brunswick has a number of theaters, including the State Theater
(908-247-7200), the George Street Playhouse (908-246-7717), and the
Crossroads Theater (908-249-5560).
The New Jersey shore is less than an hour from New Brunswick. Points
along the shore vary greatly in character. Some, such as Point
Pleasant, have long boardwalks with amusement park rides, video
arcades, etc. Others, such as Spring Lake, are quiet and
uncommercialized with clean and very pretty beaches. Further south,
about two hours from New Brunswick, are the casinos of Atlantic City.
You can walk for miles and miles along the towpath of the peaceful
Delaware and Raritan Canal which runs from New Brunswick south past
Princeton.
Your registration packet will include a pass for access to the College
Avenue Gymnasium (near the dormitories, #77 on map).
FURTHER INFORMATION. If you have any questions or problems, please
send email to colt94@research.att.com or to ml94@cs.rutgers.edu.
A map of the campus, abstracts of workshops/tutorials, updates of this
announcement, and other information will be available via anonymous
ftp from cs.rutgers.edu in the directory pub/learning94.
For New Jersey Transit fare and schedule information, call
800-772-2222 (in New Jersey) or 201-762-5100 (out-of-state).
TECHNICAL PROGRAM
The technical program for the conferences has not yet been finalized,
but will be distributed sometime in April. All ML technical sessions
will be held July 11-13, and all COLT sessions will be held July
12-15.
INVITED LECTURES:
* Michael Jordan, "Hidden decision tree models."
* Stephen Muggleton, "Recent advances in inductive logic
programming."
* Fernando Pereira, "Frequencies vs biases: Machine learning
problems in natural language processing."
PAPERS ACCEPTED TO ML:
A Baysian framework to integrate symbolic and neural learning. Irina
Tchoumatchenko, Jean Gabriel Ganascia.
A case for Occam's razor in the task of rule-base refinement. J.
Jeffrey Mahoney, Raymond Mooney.
A conservation law for generalization performance. Cullen Schaffer.
A constraint-based induction algorithm in FOL. Michele Sebag.
A Modular Q-learning architecture for manipulator task decomposition.
Chen Tham, Richard Prager.
A new method for predicting protein secondary structures based on
stochastic tree grammars. Naoki Abe, Hiroshi Mamitsuka.
A powerful heuristic for the discovery of complex patterned behavior.
Raul E. Valdes-Perez, Aurora Perez.
An efficient subsumption algorithm for inductive logic programming.
Jorg-Uwe Kietz, Marcus Lubbe.
An improved algorithm for incremental induction of decision trees.
Paul Utgoff.
An incremental learning approach for completable planning. Melinda T.
Gervasio, Gerald F. DeJong.
Combining top-down and bottom-up techniques in inductive logic
programming. John M. Zelle, Raymond Mooney, Joshua Konvisser.
Comparison of boosting to other ensemble methods using neural
networks. Harris Drucker, Yann LeCun, L. Jackel, Corinna Cortes,
Vladimir Vapnik.
Compositional instance-based learning. Karl Branting, Patrick Broos.
Consideration of risk in reinforcement learning. Matthias Heger.
Efficient algorithms for minimizing cross validation error. Mary Lee,
Andrew W. Moore.
Exploiting the ordering of observed problem-solving
steps for knowledge base refinement: an apprenticeship approach.
Steven Donoho, David C. Wilkins.
Getting the most from flawed theories. Moshe Koppel, Alberto Segre,
Ronen Feldman.
Greedy attribute selection. Richard A. Caruana, Dayne Freitag.
Hierarchical self-organization in genetic programming. Justinian
Rosca, Dana Ballard.
Heterogeneous uncertainty sampling for supervised learning. David D.
Lewis, Jason Catlett.
Improving accuracy of incorrect domain theories. Lars Asker.
In defense of C4.5: notes on learning one-level decision trees. Tapio
Elomaa.
Increasing the efficiency of simulated annealing search by learning to
recognize (un)promising runs. Yoichihro Nakakuki, Norman Sadeh.
Incremental multi-step Q-learning. Jing Peng, Ronald Williams.
Incremental reduced error pruning. Johannes Furnkranz, Gerhard
Widmer.
Irrelevant features and the subset selection problem. George H. John,
Ron Kohavi, Karl Pfleger.
Learning by experimentation: incremental refinement of incomplete
planning domains. Yolanda Gil.
Learning disjunctive concepts by means of genetic algorithms. Attilio
Giordana, Lorenza Saitta, F. Zini.
Learning recursive relations with randomly selected small training
sets. David W. Aha, Stephane Lapointe, Charles Ling, Stan Matwin.
Learning semantic rules for query reformulation. Chun-Nan Hsu, Craig
Knoblock.
Markov games as a framework for multi-agent reinforcement learning.
Michael Littman.
Model-Free reinforcement learning for non-markovian decision problems.
Satinder Pal Singh, Tommi Jaakkola, Michael I. Jordan.
On the worst-case analysis of temporal-difference learning algorithms.
Robert Schapire, Manfred Warmuth.
Prototype and feature selection by sampling and random mutation hill
climbing algorithms. David B. Skalak.
Reducing misclassification costs: Knowledge-intensive approaches to
learning from noisy data. Michael J. Pazzani, Christopher Merz,
Patrick M. Murphy, Kamal M. Ali, Timothy Hume, Clifford Brunk.
Revision of production system rule-bases. Patrick M. Murphy, Michael
J. Pazzani.
Reward functions for accelerated learning. Maja Mataric.
Selective reformulation of examples in concept learning. Jean-Daniel
Zucker, Jean Gabriel Ganascia.
Small sample decision tree pruning. Sholom Weiss, Nitin Indurkhya.
The generate, test and explain discovery system architecture. Michael
de la Maza.
The minimum description length principle and categorical theories. J.
R. Quinlan.
To discount or not to discount in reinforcement learning: a case
study comparing R~learning and Q~learning. Sridhar Mahadevan.
Towards a better understanding of memory-based and Bayesian
classifiers. John Rachlin, Simon Kasif, Steven Salzberg, David W.
Aha.
Using genetic search to refine knowledge-based neural networks. David
W. Opitz, Jude Shavlik.
Using sampling and queries to extract rules from trained neural
networks. Mark W. Craven, Jude Shavlik.
WORKSHOPS AND DIMACS-SPONSORED TUTORIALS
On Sunday, July 10, we are pleased to present four all-day workshops,
five half-day tutorials, and one full-day advanced tutorial. The
DIMACS-sponsored tutorials are free and open to the general public.
Participation in the workshops is also free, but is at the discretion
of the workshop organizers. Note that some of the workshops have
quickly approaching application deadlines. Please contact the
workshop organizers directly for further information. Some
information is also available on our ftp site (see "further
information" above).
TUTORIALS:
T1. State of the art in learning DNF rules morning/afternoon
(advanced tutorial)
Dan Roth danr@das.harvard.edu
Jason Catlett catlett@research.att.com
T2. Descriptional complexity and inductive learning morning
Ed Pednault epdp@research.att.com
T3. Computational learning theory: introduction and survey morning
Lenny Pitt pitt@cs.uiuc.edu
T4. What does statistical physics have to say about learning? morning
Sebastian Seung seung@physics.att.com
Michael Kearns mkearns@research.att.com
T5. Reinforcement learning afternoon
Leslie Kaelbling lpk@cs.brown.edu
T6. Connectionist supervised learning--an engineering afternoon
approach
Tom Dietterich tgd@research.cs.orst.edu
Andreas Weigend andreas@cs.colorado.edu
WORKSHOPS:
W1. Robot Learning morning/afternoon/evening
Sridhar Mahadevan mahadeva@csee.usf.edu
W2. Applications of descriptional complexity to afternoon/evening
inductive, statistical and visual inference
Ed Pednault epdp@research.att.com
W3. Constructive induction and change of morning/afternoon
representation
Tom Fawcett fawcett@nynexst.com
W4. Computational biology and machine learning morning/afternoon
Mick Noordewier noordewi@cs.rutgers.edu
Lindley Darden darden@umiacs.umd.edu
REGISTRATION FOR COLT94/ML94
Please complete the registration form below, and mail it with your
payment for the full amount to:
Priscilla Rasmussen, ML/COLT'94
Rutgers, The State University of NJ
Laboratory for Computer Science Research
Hill Center, Busch Campus
Piscataway, NJ 08855
(Sorry, registration cannot be made by email, phone or fax.) Make
your check or money order payable in U.S. dollars to Rutgers
University. For early registration, and to request dorm housing, this
form must be mailed by May 27, 1994. For questions about
registration, please contact Priscilla Rasmussen
(rasmussen@cs.rutgers.edu; 908-932-2768).
Name: _____________________________________________________
Affiliation: ______________________________________________
Address: __________________________________________________
___________________________________________________________
Country: __________________________________________________
Phone: _______________________ Fax: _______________________
Email: ____________________________________________________
Confirmation will be sent to you by email.
REGISTRATION. Please circle the *one* conference for which you are
registering. (Even if you are planning to attend both conferences,
please indicate the one conference that you consider to be "primary.")
COLT94 ML94
The registration fee includes a copy of the proceedings for the *one*
conference circled above (extra proceedings can be ordered below).
Also included is admission to all ML94 and COLT94 talks and events
(except that student registration does not include a banquet ticket).
Regular advance registration: $190 $_______
ACM/SIG member advance registration: $175 $_______
Late registration (after May 27): $230 $_______
Student advance registration: $85 $_______
Student late registration (after May 27): $110 $_______
Extra reception tickets (July 12): _____ x $17 = _______
Extra banquet tickets (July 13): _____ x $40 = _______
Extra COLT proceedings: _____ x $35 = _______
Extra ML proceedings: _____ x $35 = _______
Dorm housing (from below): $_______
TOTAL ENCLOSED: $_______
How many in your party have dietary restrictions?
Vegetarian: _____ Kosher: _____ Other: ______________
Circle your shirt size: small medium large X-large
HOUSING. Please indicate your housing preference below. Descriptions
of the dorms are given under "housing" above. Dorm assignments will
be made on a first come, first served basis, so please send your
request in as early as possible. We will notify you by email if we
cannot fill your request.
_____ Check here if you plan to stay at the Hyatt (reservations must
be made directly with the hotel by June 10).
_____ Check here if you plan to make your own housing arrangements
(other than at the Hyatt).
_____ Check here to request a room in the dorms and circle the
appropriate dollar amount below:
Dorm: Stonier Campbell
Length of stay: dbl. sing. dbl.
ML only (July 9-13): $144 144 108
COLT only (July 11-15): 144 144 108
ML and COLT (July 9-15): 216 216 162
If staying in a double in the dorms, who will your roommate
be? ____________________________________
For either dorm, please indicate expected day and time of arrival and
departure. Note that check-in for the dorms must take place between
4pm and midnight on July 9-13.
Expected arrival: ______ ______
(date) (time)
Expected departure: ______ ______
(date) (time)
TUTORIALS. The DIMACS-sponsored tutorials on July 10 are free and
open to the general public. For our planning purposes, please circle
those tutorials you plan to attend.
Morning: T1 T2 T3 T4
Afternoon: T1 T5 T6
To participate in a workshop, please contact the workshop organizer
directly. There is no fee for any workshop, and all workshops will be
held on July 10.
REFUNDS. The entire dorm fee, and one-half of the registration fee
are refundable through June 24. Send all requests by email to
rasmussen@cs.rutgers.edu.
------------------------------
Subject: Second Call for Papers
Date: Fri, 18 Mar 1994 17:21:43 +0900
From: Hiroki Ishizaka <ishizaka@donald.ai.kyutech.ac.jp>
*** CALL FOR PAPERS ***
ALT'94
Fifth International Workshop on Algorithmic Learning Theory
Reinhardsbrunn Castle, Germany
October 13-15, 1994
The Fifth International Workshop on Algorithmic Learning Theory (ALT'94)
will be held at the Reinhardsbrunn Castle, Friedrichroda, Germany during
October 13-15, 1994. The workshop will be supported by the German Computer
Science Society (GI) in cooperation with the Japanese Society for
Artificial Intelligence (JSAI) and it will be coupled with the Fourth
International Workshop on Analogical and Inductive Inference for Program
Synthesis (AII'94), which will be held October 10-11. We invite
submissions to ALT'94 from researchers in algorithmic learning or its
related fields, such as (but not limited to) the theory of machine
learning, computational logic of/for machine discovery, inductive
inference, query learning, learning by analogy, neural networks, pattern
recognition, and applications to databases, gene analysis, etc. The
conference will include presentations of refereed papers and invited talks
by Dr. Naoki Abe from NEC, Prof. Michael M. Richter from Kaiserslautern and
Prof. Carl H. Smith from Maryland.
SUBMISSION. Authors must submit six copies of their extended abstracts to :
Prof. Setsuo Arikawa - ALT'94
RIFIS, Kyushu University 33
Fukuoka 812, Japan
Abstracts must be received by
April 15, 1994.
Notification of acceptance or rejection will be mailed to the first (or
designated) author by June 1, 1994.
Camera-ready copy of accepted papers will be due July 4, 1994.
FORMAT. The submitted abstract should consist of a cover page with
title, authors' names, postal and e-mail addresses, and an
approximately 200 word summary , and a body not longer than ten (10)
pages of size A4 or 7x10.5 inches in twelve-point font. Papers not
adhering to this format may be returned without review.
Double-sided printing is strongly encouraged.
POLICY. Each submitted abstract will be reviewed by at least four
members of the program committee, and be judged on clarity,
significance, and originality. Submissions should contain new
results that have not been published previously. Submissions to
ALT'94 may be submitted to AII'94, but if so a statement to this
effect must appear on the cover page or the first page.
Proceedings will be published as a volume in the Lecture Notes Series
in Artificial Intelligence from Springer-Verlag, and some selected
papers will be included in a special issue of the Annals of Mathematics
and Artificial Intelligence.
For more information, contact :
ALT94@informatik.th-leipzig.de
alt94@rifis.sci.kyushu-u.ac.jp
CONFERENCE CHAIR :
K.P. Jantke
HTWK Leipzig (FH)
Fachbereich IMN
Postfach 66
04251 Leipzig, Germany
janos@informatik.th-leipzig.de
PROGRAM COMMITTEE CHAIR :
Setsuo Arikawa, Kyushu Univ.
alt94@rifis.sci.kyushu-u.ac.jp
PROGRAM COMMITTEE :
N. Abe (NEC), D. Angluin (Yale U.), J. Barzdins (U.Latvia),
A. Biermann (Duke U.), J. Case (U.Delaware), R. Daley (U.Pittsburgh),
P. Flach (Tilburg U.), R. Freivalds (U.Latvia), M. Haraguchi (TiTech),
H. Imai(U.Tokyo), B. Indurkhya (Northeastern U.), P. Laird (NASA),
Y. Kodratoff (U.Paris-Sud), A. Maruoka (Tohoku U.),
S. Miyano (Kyushu U.), H. Motoda (Hitachi), S. Muggleton (Oxford U.),
M. Numao (TiTech), L. Pitt (U. Illinois),
Y. Sakakibara (Fujitsu Lab.), P. Schmitt (U. Karlsruhe),
T. Shinohara (KIT), C. Smith (U.Maryland), E. Ukkonen (U.Helsinki),
O. Watanabe (TiTech), R. Wiehagen (U.Kaiserslautern),
T. Yokomori (U.Electro-Comm.), T. Zeugmann (TH Darmstadt),
LOCAL ARRANGEMENTS COMMITTEE CHAIR :
Erwin Keusch
HTWK Leipzig (FH)
Fachbereich IMN
Postfach 66
04251 Leipzig, Germany
erwin@informatik.th-leipzig.de
------------------------------
Date: Wed, 23 Mar 1994 10:54:58 +0700
From: "Peter A. Flach" <Peter.Flach@kub.nl>
Subject: NEW BOOK: Simply Logical - Intelligent Reasoning by Example
The following book is now available. For interested readers with access to the
World Wide Web, more information can be obtained at the URL
http://machtig.kub.nl:2080/Infolab/Peter/SimplyLogical.html
SIMPLY LOGICAL
Intelligent Reasoning by Example
Peter Flach, Tilburg University, the Netherlands
256 pp., March 1994, John Wiley & Sons
0471 94152 2 (paperback) appr. $31.95
0471 94215 4 (book+disk) appr. $44.00
0471 94153 0 (disk only) appr. $30.00
BLURB
This book deals with methods to implement intelligent reasoning by means of
Prolog programs. It is written from the shared viewpoints of Computational Logic
and Artificial Intelligence, covering the theoretical and practical aspects of
Prolog programming as well as topics such as knowledge representation, search
techniques, and heuristics. Advanced reasoning techniques presented in the final
part of the book include abduction, default reasoning, and induction. Most of
these techniques draw upon recent journal articles and conference papers,
references to which are included. The book is aimed at researchers,
practitioners and students (advanced undergraduate or graduate level) in
Artificial Intelligence and Logic Programming. It can be used as a textbook for
a graduate or advanced undergraduate course in either of these fields. Apart
from a basic knowledge of logic and some familiarity with computer programming,
no prior knowledge is assumed.
From the Foreword by Professor Robert Kowalski:
"This book by Peter Flach is an important addition to the many excellent books
covering aspects of Logic Programming, filling a gap both by including new
material on abduction and Inductive Logic Programming and by relating Logic
Programming theory and Prolog programming practice in a sound and convincing
manner. It relieves me of the temptation to write a revised edition of my own
book, Logic for Problem Solving."
TABLE OF CONTENTS
Foreword ix
Preface xi
Acknowledgements xvi
I Logic and Logic Programming 1
1. A brief introduction to clausal logic 3
1.1 Answering queries 5
1.2 Recursion 7
1.3 Structured terms 11
1.4 What else is there to know about clausal logic? 15
2. Clausal logic and resolution: theoretical backgrounds 17
2.1 Propositional clausal logic 18
2.2 Relational clausal logic 25
2.3 Full clausal logic 30
2.4 Definite clause logic 35
2.5 The relation between clausal logic and Predicate Logic 38
Further reading 41
3. Logic Programming and Prolog 43
3.1 SLD-resolution 44
3.2 Pruning the search by means of cut 47
3.3 Negation as failure 52
3.4 Other uses of cut 58
3.5 Arithmetic expressions 60
3.6 Accumulators 63
3.7 Second-order predicates 66
3.8 Meta-programs 68
3.9 A methodology of Prolog programming 74
Further reading 77
II Reasoning with structured knowledge 79
4. Representing structured knowledge 83
4.1 Trees as terms 84
4.2 Graphs generated by a predicate 88
4.3 Inheritance hierarchies 90
Further reading 97
5. Searching graphs 99
5.1 A general search procedure 99
5.2 Depth-first search 103
5.3 Breadth-first search 106
5.4 Forward chaining 110
Further reading 115
6. Informed search 117
6.1 Best-first search 117
6.2 Optimal best-first search 124
6.3 Non-exhaustive informed search 127
Further reading 128
III Advanced reasoning techniques 129
7. Reasoning with natural language 131
7.1 Grammars and parsing 132
7.2 Definite Clause Grammars 134
7.3 Interpretation of natural language 139
Further reading 145
8. Reasoning with incomplete information 147
8.1 Default reasoning 148
8.2 The semantics of incomplete information 154
8.3 Abduction and diagnostic reasoning 159
8.4 The complete picture 166
Further reading 169
9. Inductive reasoning 171
9.1 Generalisation and specialisation 173
9.2 Bottom-up induction 178
9.3 Top-down induction 184
Further reading 191
Appendices 193
A. A catalogue of useful predicates 195
A.1 Built-in predicates 195
A.2 A library of utility predicates 197
B. Two programs for logical conversion 201
B.1 From Predicate Logic to clausal logic 201
B.2 Predicate Completion 206
C. Answers to selected exercises 211
Index 232
_____________________________________________________________________________
For ordering information, contact
Nikki Phillips
John Wiley & Sons Ltd
Baffins Lane
Chicester, West Sussex, PO19 1UD
United Kingdom
Telephone: +44 243 779777
Fax: +44 243 775878
_________________________________+________________________________________
Peter.Flach@kub.nl ++ Institute for Language Technology
+ + and Artificial Intelligence
Telephone: +31 13 663119 + + Tilburg University, POBox 90153
Facsimile: +31 13 663069 +++ 5000 LE Tilburg, the Netherlands
_______________________________+++________________________________________
<A HREF=http://machtig.kub.nl:2080/Infolab/Peter/Peter.html>More info!</A>
------------------------------
Date: Wed, 30 Mar 94 10:25:17 CST
From: Wei-Min Shen <wshen@mcc.com>
Subject: AUTONOMOUS LEARNING FROM THE ENVIRONMENT
*****************************************************
* Wei-Min Shen *
* AUTONOMOUS LEARNING FROM THE ENVIRONMENT *
* Foreword by Herbert A. Simon *
* Computer Science Press, W.H. Freeman and Company *
* March 1994, 355 pp. *
* ISBN 0-7167-8265-0 *
* Hardcover $49.00 *
*****************************************************
BLURB:
------
As the fields of Artificial Intelligence and Robotics are being
revolutionized by advances in autonomous learning, a conceptual
framework for addressing the general problem of learning from the
environment has been sorely lacking --- until now. AUTONOMOUS
LEARNING FROM THE ENVIRONMENT provides a principled model that
integrates once disparate aspects of this burgeoning field and
facilitates systematic analysis of present and future autonomous
learning systems.
This unique work presents a unified conception of environmental
model abstraction, as well as the scientific foundations and
practical applications of autonomous learning systems. Drawing on
principles of human cognition, it proposes a percept and action
based mechanism that enables autonomous systems to continually
analyze and adapt to their environment in achieving their goals,
hence improving their performance without reprogramming by human
intervention.
Combining state-of-the-art theory with illustrative examples and
applications, AUTONOMOUS LEARNING FROM THE ENVIRONMENT provides
clear, up-to-date coverage of three essential tasks of developing
an autonomous learning system: active model abstraction, modal
application, and integration. It also contains an implemented
system LIVE that autonomously interacts with its environment to
solve problems and discover new concepts; readers may use many
algorithms in the book to generate their own simulations.
A pathbreaking addition to the professional literature,
AUTONOMOUS LEARNING FROM THE ENVIRONMENT is an ideal
supplementary text for Artificial Intelligence and Robotics
courses and an essential book for any computer science
library. As Herbert A. Simon states in the foreword: ``Most
readers, I think, will experience more than one surprise as they
explore the pages of this book, ...... [it] has provided us with
an indispensable vade mecum for our explorations of systems that
learn from their environments.''
TABLE OF CONTENTS:
Foreword by Herbert A. Simon
Preface
1 Introduction
1.1 Why Autonomous Learning Systems?
1.2 What Is Autonomous Learning?
1.3 Approaches to Autonomous Learning
1.4 What Is in This Book?
2 The Basic Definitions
2.1 Learning from the Environment
2.2 The Learner and Its Actions, Percepts, Goals and Models
2.3 The Environment and Its Types
2.3.1 Transparent Environment
2.3.2 Translucent Environment
2.3.3 Uncertain Environment
2.3.4 Semicontrollable Environment
2.4 Examples of Learning from the Environment
2.5 Summary
3 The Tasks of Autonomous Learning
3.1 Model Abstraction
3.1.1 The Choice of Model Forms
3.1.2 The Evaluation of Models
3.1.3 The Revision of Models
3.1.4 Active and Incremental Approximation
3.2 Model Application
3.3 Integration: The Coherent Control
3.4 Views from Other Scientific Disciplines
3.4.1 Function Approximation
3.4.2 Function Optimization
3.4.3 Classification and Clustering
3.4.4 Inductive Inference and System Identification
3.4.5 Learning Finite State Machines and Hidden Markov Models
3.4.6 Dynamic Systems and Chaos
3.4.7 Problem Solving and Decision Making
3.4.8 Reinforcement Learning
3.4.9 Adaptive Control
3.4.10 Developmental Psychology
3.5 Summary
4 Model Abstraction in Transparent Environments
4.1 Experience Spaces and Model Spaces
4.2 Model Construction via Direct Recording
4.3 Model Abstraction via Concept Learning
4.4 Aspects of Concept Learning
4.4.1 The Partial Order of Models
4.4.2 Inductive Biases: Attribute Based and Structured
4.4.3 Correctness Criteria: PAC and Others
4.5 Learning from Attribute-Based Instances and Related Algorithms
4.6 Learning from Structured Instances: The FOIL Algorithm
4.7 Complementary Discrimination Learning (CDL)
4.7.1 The Framework of Predict-Surprise-Identify-Revise
4.8 Using CDL to Learn Boolean Concepts
4.8.1 The CDL1 Algorithm
4.8.2 Experiments and Analysis
4.9 Using CDL to Learn Decision Lists
4.9.1 The CDL2 Algorithm
4.9.2 Experiments and Analysis
4.10 Using CDL to Learn Concepts from Structured Instances
4.11 Model Abstraction by
Neural Networks
4.12 Bayesian Model Abstraction
4.12.1 Concept Learning
4.12.2 Automatic Data Classification
4.12.3 Certainty Grids
4.13 Summary
5 Model Abstraction in Translucent Environments
5.1 The Problems of Construction and Synchronization
5.2 The L* Algorithm for Learning Finite Automata
5.3 Synchronizing L* by Homing Sequences
5.4 The CDL+ Framework
5.5 Local Distinguishing Experiments (LDEs)
5.6 Model Construction with LDEs
5.6.1 Surprise
5.6.2 Identify and Split
5.6.3 Model Revision
5.7 The CDL+1 Learning Algorithm
5.7.1 Synchronization by LDEs
5.7.2 Examples and Analysis
5.8 Discovering Hidden Features When Learning Prediction Rules
5.9 Stochastic Learning Automata
5.10 Hidden Markov Models
5.10.1 The Forward and Backward Procedures
5.10.2 Optimizing Model Parameters
5.11 Summary
6 Model Application
6.1 Searching for Optimal Solutions
6.1.1 Dynamic Programming
6.1.2 The A* Algorithms
6.1.3 Q-Learning
6.2 Searching for Satisficing Solutions
6.2.1 The Real-Time A* Algorithm
6.2.2 Means--Ends Analysis
6.2.3 Distal Supervised Learning
6.3 Applying Models to Predictions and Control
6.4 Designing and Learning from Experiments
6.5 Summary
7 Integration
7.1 Integrating Model Construction and Model Application
7.1.1 Transparent Environments
7.1.2 Translucent Environments
7.2 Integrating Model Abstraction and Model Application
7.2.1 Transparent Environments
7.2.1.1 Distal Learning with Neural Networks
7.2.1.2 Integrating Q-Learning with Generalization
7.2.1.3 Integration via Prediction Sequence
7.2.2 Translucent Environments
7.2.2.1 Integration Using the CDL+ Framework
7.3 Summary
8 The LIVE System
8.1 System Architecture
8.2 Prediction Rules: The Model Representation
8.3 LIVE's Model Description Language
8.3.1 The Syntax
8.3.2 The Interpretation
8.3.3 Matching an Expression to a State
8.4 LIVE's Model Application
8.4.1 Functionality
8.4.2 An Example of Problem Solving
8.4.3 Some Built-in Knowledge for Controlling the Search
8.5 Summary
9 Model Construction through Exploration
9.1 How LIVE Creates Rules from Objects' Relations
9.2 How LIVE Creates Rules from Objects' Features
9.2.1 Constructing Relations from Features
9.2.2 Correlating Actions with Features
9.3 How LIVE Explores the Environment
9.3.1 The Explorative Plan
9.3.2 Heuristics for Generating Explorative Plans
9.4 Discussion
10 Model Abstraction with Experimentation
10.1 The Challenges
10.2 How LIVE Revises Its Rules
10.2.1 Applying CDL to Rule Revision
10.2.2 Explaining Surprises in the Inner Circles
10.2.3 Explaining Surprises in the Outer Circles
10.2.4 Defining New Relations for Explanations
10.2.5 When Overly Specific Rules Are Learned
10.3 Experimentation: Seeking Surprises
10.3.1 Detecting Faulty Rules during Planning
10.3.2 What Is an Experiment?
10.3.3 Experiment Design and Execution
10.3.4 Related Work on Learning from Experiments
10.4 Comparison with Previous Rule-Learning Methods
10.5 Discussion
11 Discovering Hidden Features
11.1 What Are Hidden Features?
11.2 How LIVE Discovers Hidden Features
11.3 Using Existing Functions and Terms
11.4 Using Actions as Well as Percepts
11.5 The Recursive Nature of Theoretical Terms
11.6 Comparison with Other Discovery Systems
11.6.1 Closed-Eye versus Open-Eye Discovery
11.6.2 Discrimination and the STABB System
11.6.3 LIVE as an Integrated Discovery System
12 LIVE's Performance
12.1 LIVE as LOGIC1
12.1.1 Experiments with Different Goals
12.1.2 Experiments with Different Explorations
12.1.3 Experiments with Different Numbers of Disks
12.2 LIVE as LOGIC2 (Translucent Environments)
12.3 LIVE on the Balance Beam
12.3.1 Experiments with Training Instances in Different Orders
12.3.2 Experiments with the Constructors in Different Orders
12.3.3 Experiments with Larger Sets of Constructors
12.4 LIVE's Discovery in Action-Dependent Environments
12.5 LIVE as a HAND-EYE Learner
12.6 Discussion
13 The Future of Autonomous Learning
13.1 The Gap Between Interface and Cognition
13.2 Forever Being Human's Friends
Appendix A: The Implementations of the Environments
Appendix B: LIVE's Running Trace
Bibliography
Index
ORDERING INFORMATION:
To order, please contact your local book stores or Computer Science
Press, W.H. Freeman, at the following addresses:
USA:
Call toll-free 1-800-877-5351
CSP, W.H. Freeman and Company
41 Madison Avenue
New York, NY 10010
212-576-9400
CSP, W.H. Freeman and Company
4419 W. 1980, S.
Salt Lake City, UT 84104
801-973-4660
England:
Liz Warner
W.H. Freeman and Company, Limited
20 Beaumont Street
Oxford, OX1 2NQ
England
Telephone: 011.44.865.726.975
Fax: 011.44.865.790.391
If you are seriously interested in using the text in the course,
contact Editor for Computer Science Press, Burt Gabriel, for a
complimentary copy.
Burt Gabriel
Editor, Computer Science Press
W.H. Freeman and Company
41 Madison Avenue
New York, NY 10010
212-576-9400
email: 0005387898@mcimail.com
------------------------------
End of ML-LIST (Digest format)
****************************************